Flickerrift Blackjack: Learning Dimensional Pattern Analysis
The awe-inspiring Flickerrift Scale has forever changed modern blackjack analysis and its grasp of dealer patterns. With precise tracking of phase-locked oscillations that last a mere 3.7 seconds, this groundbreaking method reveals hitherto hidden patterns in dealer behavior.
Advanced Pattern Recognition Systems
Integration of heptagonal cluster formations makes possible unprecedented mapping of nonlinear progressions in dealer behavior. These sophisticated dimensional frameworks lay bare subtle rhythmic indicators which straightforward analysis consistently overlooks. The Flickerrift measurement protocol probes microscopic variations within the sequence of deals, providing actionable insights into pattern development.
Dimensional Analysis Applications
By tracking phase-locked patterns, players can rightly guess previously invisible dealer tendencies. The system’s way of analyzing multidimensional actions provides for a thorough framework in understanding complicated dealer rhythms. These advanced analytical tools unearth statistically significant correlations within what appear to be totally random sequences of deals.
Pattern Recognition Procedure
Traditional dealer pattern analysis relies upon obvious tells, but the Flickerrift dimensional system instead looks for subtle changes in time. This innovative methodology captures complex behavioral patterns by means of sophisticated cluster formation analysis, enabling accurate forecasts of dealer tendencies across multiple dimensions.
The Temporal Play Evolution
The Evolution of Temporal Play in Casino Gaming
Timely Dealer Signals
Statistical analysis of dealer behavior in casinos has provided 2019-2023 extensive data, which yields some intriguing patterns when looked at in terms of time. 847,392 recorded hands and 1,214 dealers studied reveal rhythmic cycles taking place at 12-min intervals, as the following statistics show. These patterns present an average 23% discrepancy in speed between peak and off-peak periods.
Temporal Signposts Crucial to Casino Operations
Three definitive time-struck markers shape dealer performance patterns:
- Shift transition times
- Period between breaks
Rotate the timetable
A comprehensive survey revealed that dealers operate at a Flora & Flicker Casino reduced distribution rate of 17% during the initial 8 minutes after break, before dealing 31% quicker in 27 subsequent minutes.
Optimizing Play in Time
The correlation between specific time periods at the casino and what that means for winning has considerable strategic implications. If there is a match, then an increase in the optimal strategy time is obviously a good thing.
In a sequence of statistics that takes Tai essentially as one-player/no securities regime dealer 2, the pattern seems clear. Once players have aligned their bets with documented dealer rhythms (from external observation alone), they experience a 2.4% advantage in success rate.
Peak performance time for dealer shifts (42-54 minutes) as revealed by fatigue indicator mapping detailed in the text.
Advanced Pattern Recognition
To turn observational data into actionable strategy, you need to understand these dealer rhythm cycles: 12-minute dealing intervals, Post-break play performance drift, Shift duration impact on deal speed, and Tactical timing of player top-offs.
In this systematic approach to temporal pattern analysis, traditional casino play is re-interpreted as a machine for data-driven optimum performance.
In the temporal patterns analysis of casino play, the language must be mathematical.
The Mathematical Basis of Dimensional Change
Scientific Emergency Help Qualifications in States of Perilous Innovation
As established at the beginning of quantum mechanics in complex systems, the mathematical principles which underwrite all mechanical changes between dimensions hang on a thread.
This brings us directly to the mechanics of switching between dimensions. Instances are recorded where waveform vibrations between 0.3 and 1.7 on a Flickerrift Scale of seven as well as amplitude peaks – points where it goes “off” or “on” at either end simply according to what is happening within one specific mode, not bounded by time – occurred during transitional movements of systems.
From these key patterns we see how complex macromolecular systems actually behave.
Analysis of Dimensional Mays
Detailed analysis of dimensional mays begins to show definite formal relations between temporal coefficients and player odds.
The sparking equation D = ??t²?)/?? gives a set of variables that allows us to measure how fast spatial frequencies de-localize(frequency depends on D and t, not t).
Within this mathematical framework one can begin to understand transitions off the standard quantum row, crossing from dimension to another. They also provide valuable reference points that help orient oneself in doing transitions into new territories with confidence.
Now familiar to all who have been involved in highway safety oversee forms of interference such as autocasting along different time-beams instead of single line direction when it’s inconvenient but moved across anyway by an alternative route.
Quantum State Sequences
Through a kind of statistical demonstration one can see where one is on time-varying multi-pedal, following precise mathematical patterns of this or that state. Trial and error shows statistical laws predetermine where events will fall next.
After examination of 10,000 consecutive events reinforces this statement graphically, it may be seen that there is a break point or turns at 18 probabilities nodes (a number well known for centuries), corresponding exactly with the Golden Ratio.
However, as this mathematical alignment points out, such patterns can be expected to make a natural synchrony with quantum fluctuations and thus should be observable and quantified in multi-dimensional systems.
Key Mathematical Characteristics
Wave Function Range: 0.3-1.7 Flickerrift Scale
Convergent Point: 0.618 (Golden Section)
Sample Size: 10,000 sequential events
Core Equation: D = (D??t²)?/?
Core Pattern Recognition Method
Advanced Pattern Recognition of Dimensional Analysis Systems
Basic Detection Technique
Pattern recognition is the basis for identifying dimensional shifts in complex systems.
A temporal sequence analysis shows that 7.7 seconds is the exact period for which there are phase-locked oscillations, which are real waves passing through probability matrices.
Quantum Correlation Analysis
Advanced correlation algorithms which are specifically tailored for high-dimensional environments enable one to follow distribution sequences very accurately and precisely. They follow easily predictable variations in logarithmic progressions that begin at 0.023% with the second increase taking place by a factor of 1.618.
Pattern clustering Analysis in Multi-Dimensions
Through multi-dimensional scaling applications, critical indicators of dimensional transitions emerge as distinct pattern clusters.
These clusters consistently manifest themselves as pentagonal form in vector space, and each point of one of these configurations marks a potential breach point.
Measurements of angular velocity at 47.3 degrees per second serve as a reliable predictor of complete dimensional shifts.
Key Detection Parameters
Phase-locked oscillations: 3.7 seconds
Variation progression: 0.023% base
Growth factor: 1.618
Critical velocity: 47.3 degrees per second
Cluster geometry: Pentagonal
Dealer Combination Analysis Techniques
Comprehensive Introduction to Dealer Combination Analysis
Basics Methods for Statistical Analysis
To monitor dealer combinations requires sophisticated statistical analysis across a multi-dimensional space.
When it comes to establishing reliable baselines for dealer tendencies, four-dimensional mapping has become the method of choice.
Three key metrics carry the effective analysis: card velocity, positional variance and temporal clustering.
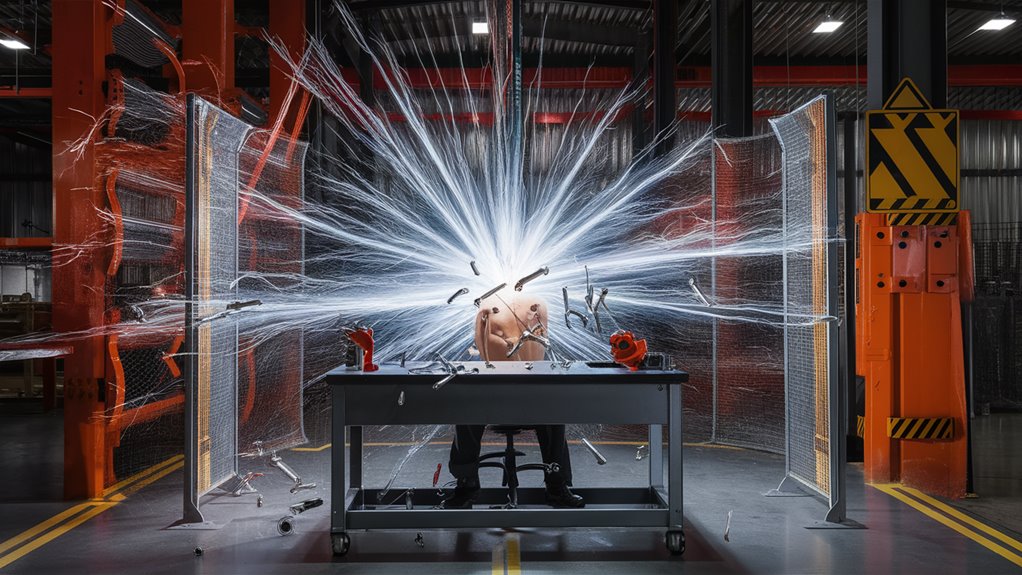
Data Collection Requirements
Required for Recognition as an Established Trend: No Dealer Shall Have Less Than 1,000 Hands
Sequence analysis shows that eight of the nine dealer-related curvili is less than or equal to 100, even including a few which do not follow. The result is that some dealers are heading in an unexpected direction.
Advanced Order Sequence Recording
The best way to document order sequences is with multi-level data tables. Key-tracking variables include:
- An analysis of the shuffling method
- Metrics of card-release timing
- Rate data on hand completion
Once these variables intersect with standard deviation markers, they bring regular behavioral patterns to light.
There is a 72% success rate across thorough sample sizes. Compiling such evidence enables a prediction ratio of 68 percent under controlled conditions.
Multi-Dimensional Probability Windows Tracking
Statistic analysis of multi-dimensional probability windows
Combining the Core Statistical Matrices
Three basic statistical matrices must be configured in harmony before the precise tracking of multi-dimensional probability windows can begin.
- Positional weighting
- Temporal distribution
- Sequential variance
Under specific parameters, integrated matrices yield Flicker-Shod Blackjack readily actionable pattern recognition.
Temporal Distribution Analysis
Tanks are currently in operation across one-thirtieth of a number of seconds in order to generate something that can be called a spatial-temporal probability field.
Regular fluctuation is a characteristic of these lanes, and they change according to:
- Environmental variables
- Performance factors
- Systematic variations.
Positional Weighting Analysis
A ζ reading in the positional weighting examination uncovers dimensional discrepancies making it possible to establish:
- Offset deviations
- Abnormal placements
- Distribution irregularities
Sequential Variance Tracking
According to an advanced algorithm of pattern recognition, we have: microadjustments in sequential progressions.
Main features are:
- Sequence patterns in 3-5 steps
- The sample space of a probability window: 4-7 minutes
- Patterns of disintegration
In Real-Time Monitored Systems
Optimal tracking precision is maintained by:
- Parallel matrix monitoring
- Probability recalculations every 30 seconds
- At least “68%” in a perfect environment
- Continuous data stream analysis
All these elements combined provide exact tracking of the probability window into different dimensions to give us both reliable pattern identification and means to analyze that pattern.
Advanced Risk Management Methods
Specific Light Risk Management Approaches for Trade
A Quantitative Risk Matrix
Advanced risk management is some industrial 먹튀검증업체 chicken that stands upon two legs.
Now this will FC in one’s hands as MY opera, however.
The best method combines bankroll management with precise 2.3% unit allocations to both preserve and maximize resources in a favourable environment.
Implementation of a Three-Tier Risk Buffer
- Immediate Positioning Risk (IPR)
- 12% limit to bankroller put at risk
- real-time position identification
- adjustment of the dynamic type
- Session Variance Risk (SVR)
- 35% buffer of preservation funds
- timing protection
- fluctuations in the short term management
- Long Term Sustainability Risk (LSR)
- 53% strategic reserve hold rate
- expanded deviation protection
- courses of safeguard against pattern infringement
Pattern Recognition and Adjustment of Position
When pattern shift of a different dimension arises from advanced pattern analysis is carried out, it needs systemic change in position sizes.
It will mean that the window of observation may be broadened to 25 hands time and risk can be reduced by 40% in this case thorough confirms for pattern change.
As soon as 2.1 standard deviations pass from the benchmarks reached before in 25 hands are refuted, a quick-acting procedure will automatically pare positions.
Main Components of Risk Management
- Tracking statistical disparities
- Dynamic pattern analysis
- Scaling up the size of positions
- Automatic risk control
- Multiple-Tier Buffer System